Why Disaggregating Data by Race is Important for Racial Equity
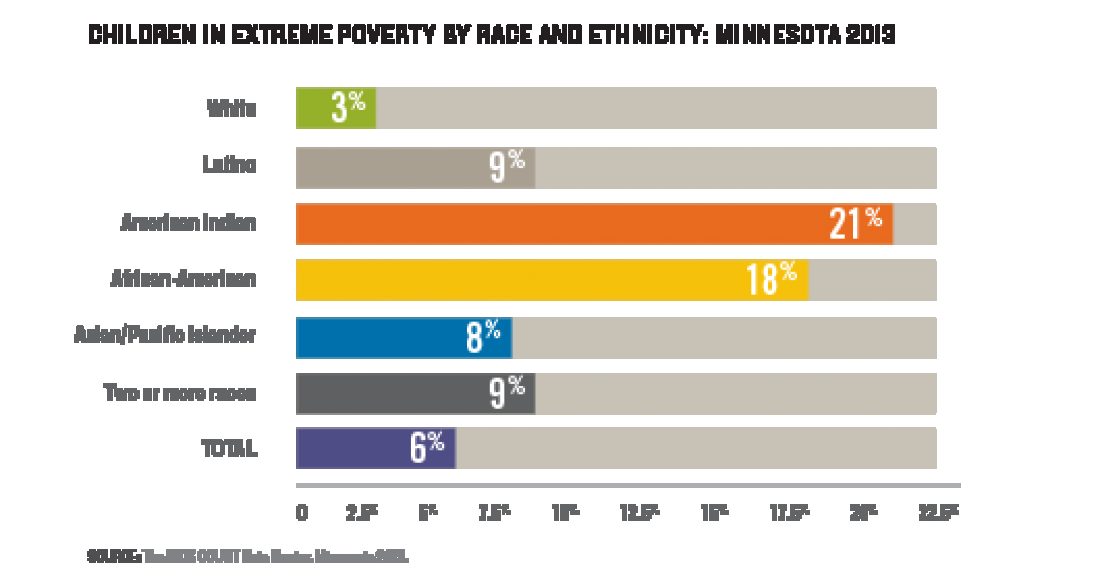
Identifying race equity problems can be challenging without the proper collection or use of data. The Foundation’s recent case study, By the Numbers: Using Disaggregated Data to Inform Policies, Practices, and Decision-Making, shows how taking apart data and presenting the information in a new way can change the way statistics look and problems are solved:
Breaking down aggregated data can uncover hidden racial inequities
The kind of data collected matters and can help unearth a problem masked by aggregate data — even data already broken down by basic racial categories. For example, consider 2010 U.S. Census Bureau statistics that showed more than half of Asian Americans had a bachelor’s degree or higher by the age of 25, the highest proportion among racial categories. Yet when the data are disaggregated to focus specifically on Southeast Asian Americans, a different picture emerges. Just 15% of Cambodian Americans, 14% of Hmong Americans, 12% of Laotian Americans and 26% of Vietnamese Americans over the age of 25 had a bachelor’s degree, the census reported. The rates for Cambodian Americans, Hmong Americans and Laotian Americans were lower than the 18% rate reported for African Americans, and the rate for Laotian Americans fell below the 13% rate reported for Latinos. As these disaggregated data show, Southeast Asian Americans experience barriers to educational attainment on par with their African-American and Latino peers, a phenomenon that could easily have been overlooked with less specific data.
Disaggregating data also helps in identifying the specifics of an established problem. The W. Haywood Burns Institute applies “REGGO”, a strategy to break down juvenile justice system data based on race, ethnicity, gender, geography and offense. When applying REGGO to Ventura County juvenile corrections data, Burns and county officials were able to document the need for reporting centers with evening tutoring or professional development in Latino communities. This intervention meant 53% fewer Latino youth admitted into detention centers. Taking data apart or even applying a strategy like REGGO to data can help reframe race equity issues and prompt targeted interventions in communities with limited resources.
The presentation of disaggregated data also matters for racial and social equity
Once data are disaggregated, presentation of data can change how people view the severity or salience of a problem. Within our case study, we highlighted the Kirwan Institute’s use of “Opportunity Mapping.” The Opportunity Mapping process gathers data related to education, health and jobs and maps them onto indicators of opportunity within communities. In so doing, the Institute situates social problems within the context of a specific community. The process culminates with a visual “heat map” that shows where opportunities are high or low in reference to population density of specific ethnic groups. The Institute found that the visual representation of social problems within the community elicited different, stronger reactions than just the presentation of raw numbers. For example, the Kirwan Institute found that Opportunity Mapping of child mortality within Ohio helped state officials see the severity of the problem.
Learn more about racial equity data collection and the importance of disaggregation
Disaggregating data and presenting it in a meaningful way can help bring attention and commitment to the solving of social and racial equity problems. For more important takeaways on why data disaggregation and a data-driven approach matter, review our full report.